Overview
The NGNS group at UC Davis has a long history of successful development of advanced testbed demonstrators for datacom and telecom networks [1-2].
Datacom (datacenter and HPC) testbeds typically consist of many photonic (off-the-shelf and research-grade) components, FPGAs, servers, and instrumentations intertwined to emulate high-performance computing systems, data centers, and computing clusters.
Telecom networking testbeds consist of elastic optical networking transceivers, wavelength selective switches RF and mmWave antennas, FPGAs, optical performance monitors, and servers, capable of emulating elastic optical networks and/or elastic RF-optical networks for 5G mm-Wave with software-defined cognitive control and management planes.
Current testbeds are continuously evolving and getting upgraded to test novel data-plane and control-plane technologies and architectures developed at UC Davis NGNS group. Of particular interest are machine-learning enhanced control plane cognitive agents equipped with observe-analyze-act algorithms to constantly optimize and adapt the interconnection network configurations in response to various phases of workloads, traffic variations, network failures, optical signals degradation, etc…
Computing and Data Center Networking Testbeds
Figure 1 shows recent HPC and Data Center testbeds developed by NGNS researchers at 2230 Kemper Hall and demoed also at the Supercomputing Conference in 2014 and 2018. These testbeds with FPGA-based emulated nodes are capable of accurately conducting clock-cycle-accurate emulations of various novel optically interconnected systems and architectures consisting of a new generation of processors, memory, and various novel optical interconnection solutions including AWGRs (LIONS, Flex-LIONS and Thin-Clos LIONS).
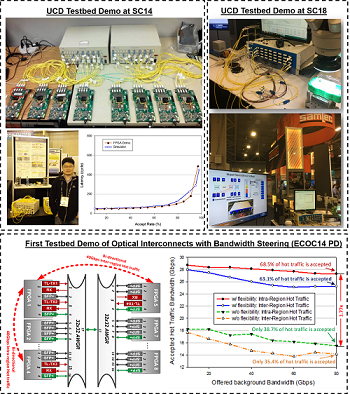
Figure 2 illustrates our most recent and ongoing testbed effort to integrate our recently developed SiPh Flex-LIONS data plane photonic interconnect solution with a cognitive control and management plane with observe-analyze-act algorithms leveraging emerging AI and machine learning tools. The testbed will include commercial top-of-rack switches, servers, and open-source software solutions for application and network control and management plane. The end goal is to demonstrate the use of innovative photonic data plane interconnect and switching solutions as well as novel cognitive control plane approaches to validate the use and benefits of photonic interconnect and switching technologies for data center and HPC applications.
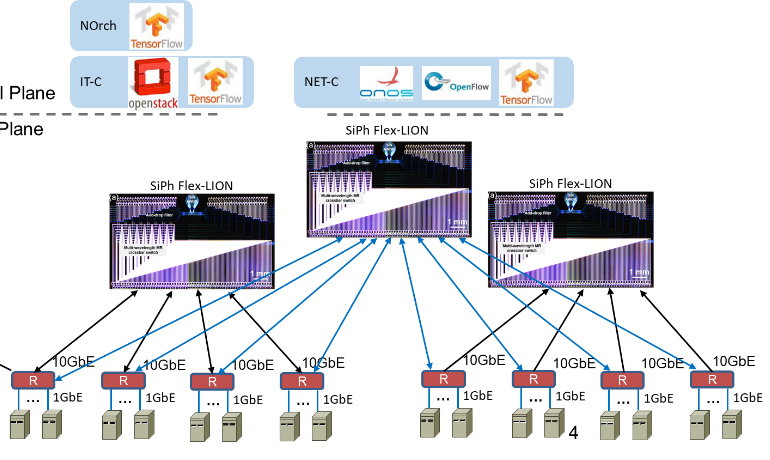
Cognitive Elastic Optical Networking Testbed
The elastic optical networking testbed at 2230 Kemper Hall is fully equipped with a management plane, control plane, and data plane where hardware and software elements are designed to support full functionality. For instance, Figure 3 illustrates broker agents and domain manager agents equipped with observe-analyze-act cognitive algorithms to control and manage the elastic optical network domains, and Figure 4 illustrates one optical networking testbed configuration for achieving the First Testbed Demonstration of Cognitive End-to-End Optical Service Provisioning with Hierarchical Learning across Multiple Autonomous Systems [3]. In the future, when these optical nodes are also equipped with RF-optical transceivers, elastic RF-optical networking (ERON), can be supported. Currently, the components are being assembled into ERON and EON testbeds with cognitive control/management planes [3-9].
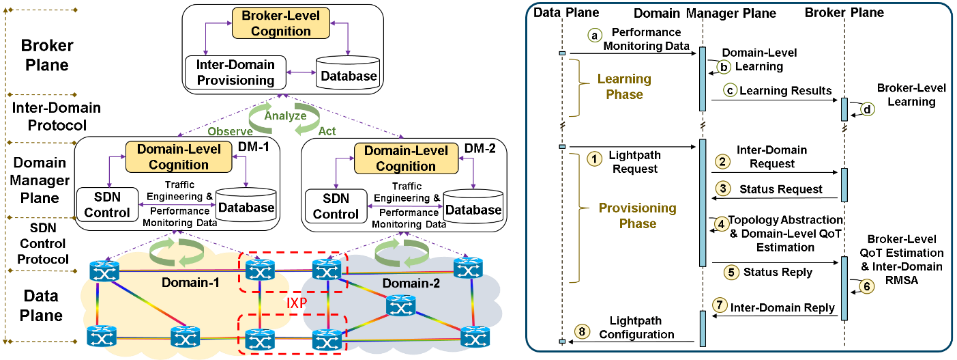
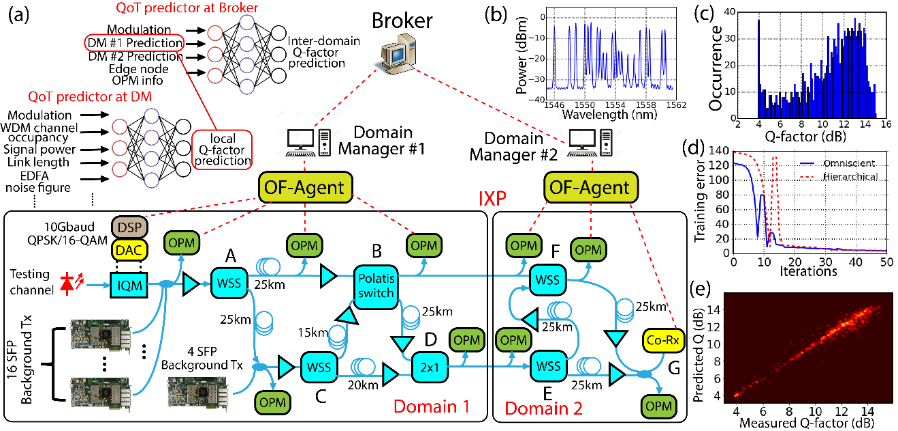
References
[1] L. Liu, W.-R. Peng, R. Casellas, T. Tsuritani, I. Morita, R. MartÃnez, R. Muñoz, M. Suzuki, and S. J. B. Yoo, “Dynamic OpenFlow-Based Lightpath Restoration in Elastic Optical Networks on the GENI Testbed,” Journal of Lightwave Technology, vol. 33, pp. 1531-1539, 2015/04/15 2015.
[2] L. Liu, W. R. Peng, R. Casellas, T. Tsuritani, I. Morita, R. MartÃnez, R. Muñoz, and S. J. B. Yoo, “Experimental demonstration of OpenFlow-based dynamic restoration in elastic optical networks on GENI testbed,” in 2014 The European Conference on Optical Communication (ECOC), 2014, pp. 1-3.
[3] G. Liu, K. Zhang, X. Chen, H. Lu, J. Guo, J. Yin, R. Proietti, Z. Zhu, and S. J. Ben Yoo, “The First Testbed Demonstration of Cognitive End-to-End Optical Service Provisioning with Hierarchical Learning across Multiple Autonomous Systems,” in Optical Fiber Communication Conference Postdeadline Papers, San Diego, California, 2018, p. Th4D.7.
[4] R. Proietti, X. Chen, K. Zhang, G. Liu, M. Shamsabardeh, A. Castro, L. Velasco, Z. Zhu, and S. J. B. Yoo, “Experimental demonstration of machine-learning-aided QoT estimation in multi-domain elastic optical networks with alien wavelengths,” IEEE/OSA Journal of Optical Communications and Networking, vol. 11, pp. A1-A10, 2019.
[5] X. Chen, B. Li, R. Proietti, Z. Zhu, and S. J. Ben Yoo, “Multi-Agent Deep Reinforcement Learning in Cognitive Inter-Domain Networking with Multi-Broker Orchestration,” in Optical Fiber Communication Conference (OFC) 2019, San Diego, California, 2019, p. M2A.2.
[6] S. J. B. Yoo, “Cognitive Heterogeneous Multi-Domain Networks with Hierarchical Learning,” in 2018 IEEE Photonics Society Summer Topical Meeting Series (SUM), 2018, pp. 1-3.
[7] R. Proietti, X. Chen, A. Castro, G. Liu, H. Lu, K. Zhang, J. Guo, Z. Zhu, L. Velasco, and S. J. B. Yoo, “Experimental Demonstration of Cognitive Provisioning and Alien Wavelength Monitoring in Multi-domain EON,” in Optical Fiber Communication Conference, San Diego, California, 2018, p. W4F.7.
[8] X. Chen, J. Guo, Z. Zhu, R. Proietti, A. Castro, and S. J. B. Yoo, “Deep-RMSA: A Deep-Reinforcement-Learning Routing, Modulation and Spectrum Assignment Agent for Elastic Optical Networks,” in 2018 Optical Fiber Communications Conference and Exposition (OFC), 2018, pp. 1-3.
[9] S. J. B. Yoo, “Multi-domain Cognitive Optical Software Defined Networks with Market-Driven Brokers,” in European Conference and Exhibition on Optical Communication (ECOC), Cannes, France, 2014, p. We.2.6.3.
[10] H. Lu, G. Liu, R. Proietti, V. Squitieri, K. Zhang, A. Castro, Q. J. Gu, Z. Ding, and S. J. Ben Yoo, “mmWave Beamforming using Photonic Signal Processing for Future 5G Mobile Systems,” in Optical Fiber Communication Conference, San Diego, California, 2018, p. M4J.3.
[11] X. Xiao, R. Proietti, G. Liu, H. Lu, P. Fotouhi, S. Werner, Y. Zhang, and S. J. B. Yoo, “Silicon Photonic Flex-LIONS for Bandwidth-Reconfigurable Optical Interconnects,” IEEE Journal of Selected Topics in Quantum Electronics, vol. 26, pp. 1-10, 2020.
[12] R. Proietti, G. Liu, X. Xiao, S. Werner, P. Fotouhi, and S. J. B. Yoo, “FlexLION: A Reconfigurable All-to-All Optical Interconnect Fabric with Bandwidth Steering,” in Conference on Lasers and Electro-Optics, San Jose, California, 2019, p. SM3G.2.
[13] R. Proietti, Y. Yin, Z. Cao, C. J. Nitta, V. Akella, and S. J. Ben Yoo, “Low-Latency Interconnect Optical Network Switch (LIONS),” in Optical Switching in Next Generation Data Centers, F. Testa and L. Pavesi, Eds., Cham: Springer International Publishing, 2018, pp. 107-127.