Overview
The rapid growth of emerging network applications and their stringent quality-of-service requirements are driving today’s backbone networks to evolve toward multi-domain elastic optical networks (MD-EONs), which can support high-capacity and user-customized end-to-end services across multiple autonomous systems (ASes) [1]. To realize resource-efficient service provisioning in MD-EONs, accurate quality-of-transmission (QoT) modeling techniques are indispensable. In this context, recent studies have reported a number of machine learning (ML)-aided cognitive QoT estimation designs that can model complex network dynamics (e.g., dynamic traffic profiles) and uncertainties (e.g., uncertain device conditions) using big data analytics [2], [3]. However, these existing works focus on single-domain scenarios and cannot be applied to MD-EONs, where only a very limited amount of domain information is available due to domain privacy concerns. Therefore, we recently proposed a hierarchical learning approach for QoT estimation in MD-EONs [4], where domain managers (DMs) work cooperatively with a broker plane by learning domain-level and inter-domain QoT estimators, respectively. Nonetheless, a major challenge remaining unmet is that the approach entails a significant amount of performance monitoring data for every inter-domain lightpath, which can be very costly and unscalable. Fortunately, the invention of transfer learning (TL) has enabled to significant reduction in the amount of effort required for training an ML task by reusing knowledge learned from relevant tasks [5]. The application of TL for QoT estimation was first studied in [6]. However, the work in [6] only adopts a very simple and straightforward TL scheme, and more importantly, does not address the challenges in MD-EONs.
Current Research
We propose Evol-TL, an evolutionary transfer learning approach, for enabling scalable QoT estimation in MD-EONs. Evol-TL exploits a broker-based MD-EON architecture, where a broker plane performs end-to-end QoT estimation by collecting encoded features from DMs. Fig. 1 depicts the schematic of Evol-TL in an MD-EON with broker orchestration and the principle of knowledge transfer in Evol-TL. Specifically, A generic algorithm (GA) is designed to enable Evol-TL to determine the proper neural network architectures and the right sets of parameters for transferring through iterative optimizations, whereas the previous works mostly rely on human experiences or brute-force searches. Evaluations with experimental data show that Evol-TL can transfer knowledge between similar tasks and significantly reduce the amount of required training data for new tasks without sacrificing the estimation accuracies.
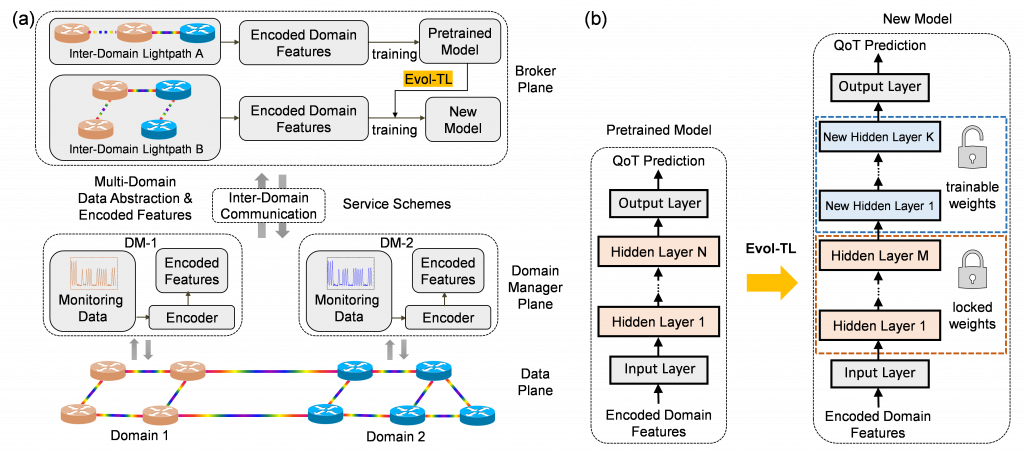
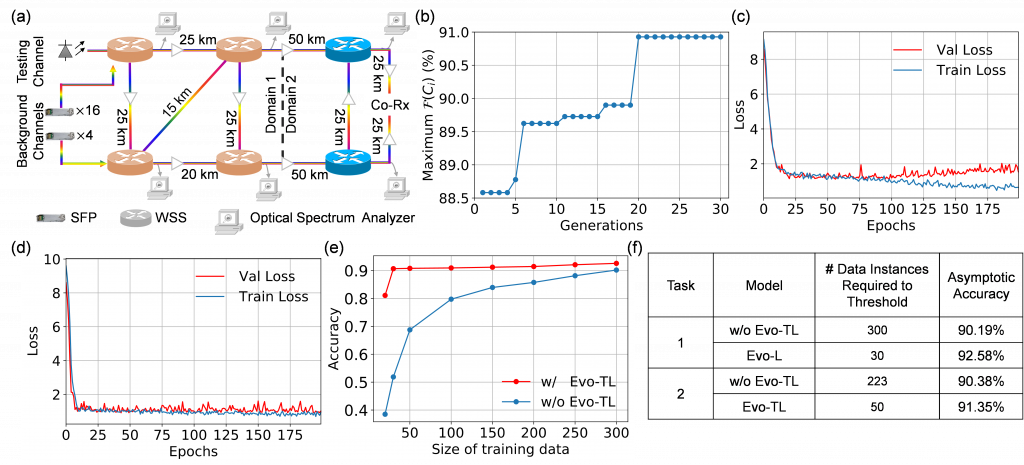
References
[1] X. Chen, R. Proietti, H. Lu, A. Castro, and S. J. B. Yoo, “Knowledge-Based Autonomous Service Provisioning in Multi-Domain Elastic Optical Networks,” in IEEE Communications Magazine, vol. 56, no. 8, pp. 152-158, August 2018.
[2] L. Barletta, A. Giusti, C. Rottondi, and M. Tornatore, “QoT Estimation for Unestablished Lighpaths using Machine Learning,” in Optical Fiber Communication Conference, OSA Technical Digest (online) (Optical Society of America, 2017), paper Th1J.1.
[3] Roberto Proietti, Xiaoliang Chen, Kaiqi Zhang, Gengchen Liu, M. Shamsabardeh, Alberto Castro, Luis Velasco, Zuqing Zhu, and S. J. Ben Yoo, “Experimental Demonstration of Machine-Learning-Aided QoT Estimation in Multi-Domain Elastic Optical Networks with Alien Wavelengths,” J. Opt. Commun. Netw. 11, A1-A10 (2019)
[4] Gengchen Liu, Kaiqi Zhang, Xiaoliang Chen, Hongbo Lu, Jiannan Guo, Jie Yin, Roberto Proietti, Zuqing Zhu, and S. J. Ben Yoo, “Hierarchical Learning for Cognitive End-to-End Service Provisioning in Multi-Domain Autonomous Optical Networks,” J. Lightwave Technol. 37, 218-225 (2019)
[5] S. J. Pan and Q. Yang, “A Survey on Transfer Learning,” in IEEE Transactions on Knowledge and Data Engineering, vol. 22, no. 10, pp. 1345-1359, Oct. 2010.
[6] W. Mo et al., “ANN-Based Transfer Learning for QoT Prediction in Real-Time Mixed Line-Rate Systems,” 2018 Optical Fiber Communications Conference and Exposition (OFC), San Diego, CA, 2018, pp. 1-3.
bookmarked!!, I love your web site!
Thanks!
Admin